The current rate of generation of high-throughput biomedical datasets is yet to be matched by our ability to extract reliable, predictive patterns from the -omics data. Despite the abundance of various high-dimensional data analysis methodologies developed to date in the biostatistics and machine learning communities, biomedical and early detection researchers still struggle to to find sensitive predictors for detecting various types of cancer and other diseases.
Our perspective on genomic and proteomic data mining emphasizes selecting a small number of key variables most essential for a given biological context. We have been working on a biomarker discovery toolbox that is conceptually based on computational physics techniques borrowed from statistical mechanics of strongly interacting systems, and integrates these methods with learning from the data.
The methodology we have developed is fairly general and is readily applicable to proteomic, genomic, and other high-throughput datasets. On the learning side, it strives to choose less variables of stronger combined predictive value, along with simpler and reliable decision boundaries (e.g. for binary classification of the type disease vs. no disease). Importantly, our methodology goes beyond the limitations of univariate variable ranking methods, building on the analogy with strongly interacting rather than uncorrelated physical systems.
The results we have obtained so far with various high-dimensional datasets highlight the advantage of using a smaller number of variables refined via careful feature selection. As an illustration, we consider a peripheral blood gene expression dataset from a European clinical study (Rotunno et al., 2011) (Affymetrix microarray data available from GEO GSE20189 and ArrayExpress E-GEOD-20189) where biomarkers were sought for early-stage detection of lung cancer. The authors identified an 8-gene predictive signature that we used as a reference point for sensitivity and specificity of cancer detection. Applying our algorithms to the same dataset, we were able to identify multiple predictive variable sets as small as 2-gene in size, which notably exceeded published performance measures. For example, the plot below illustrates a 2-gene signature that classifies lung cancer patients with specificity and sensitivity that exceed those of the 8-gene set identified in the publication.
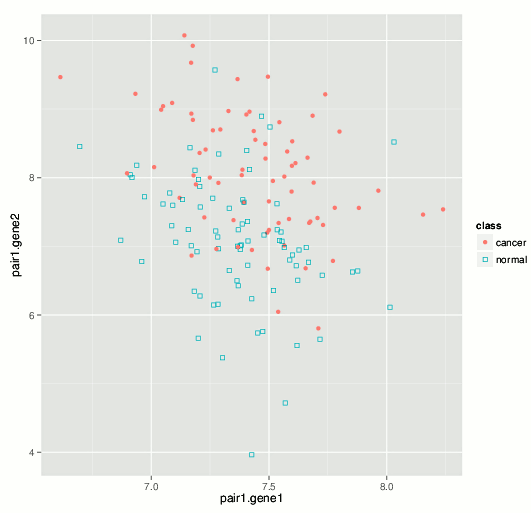
While working on various improvements and extensions of our methodology, we especially seek projects and collaborations for biomarker search in different kinds of high-throughput biological datasets. Biomarker development in the pharmaceutical target contexts are of interest as well. Please feel free to tell us about a relevant project you have in mind or refer us to potentially interested colleagues.
References
Rotunno M, Hu N, Su H, Wang C, Goldstein AM, Bergen AW, Consonni D, Pesatori AC, Bertazzi PA, Wacholder S, Shih J, Caporaso NE, Taylor PR, Landi MT. 2011. A gene expression signature from peripheral whole blood for stage I lung adenocarcinoma. Cancer prevention research (Philadelphia, Pa.) 4:1599–608. http://cancerpreventionresearch.aacrjournals.org/content/4/10/1599.